Early Career Scientist Spotlight
Dr. Adam Erickson
Computational Biosphericist
Hydrological Sciences Laboratory
What is your research focus?
I am interested in state-of-the-art computational techniques for measuring and simulating the terrestrial biosphere and biospheres in general. A primary focus of my work involves blending individual-based, landscape, and global models to create new hybrid machine-learning land models and related observation systems needed to drive these models. The long-term goal of this work is threefold: (1) to better understand our biosphere in relation to other component systems (i.e., the geosphere, hydrosphere, and atmosphere); (2) to optimize management for multiple objectives based on this understanding to achieve a sustainable biosphere (e.g., maximizing land carbon storage and albedo while minimizing biodiversity loss and greenhouse gas production under a set of economic constraints); (3) to extend these principles to synthetic biospheres and other worlds. I adopt the term biospherics in reference to the general study of biospheres, as this goal entails biology, ecology, evolution, biogeochemistry, and physics, from Earth systems to synthetic and extraterrestrial systems.
My approach to biospherics focuses on the application of modern software tools and artificial intelligence techniques (e.g., evolutionary computation, deep learning, co-processor acceleration, field robotics) to outstanding problems in the field. As a pioneer of hybrid machine-learning models of terrestrial ecosystems and deep learning in Earth system science, much of my research extends from these two lines of inquiry. I also maintain a passion for bringing the Earth sciences into the genomics and synthetic biology era, for which deep learning is essential. Each of these endeavors will require radical new measurement systems and high-fidelity models to improve global simulations in a data-driven era.
In this domain, I am most excited about our proposed robotic collection of hyperspectral and 3-D geometric information over time (i.e., 4-D spectroscopy) for monitoring the Earth surface. We call this the Hypersurface Network (formerly 5DNet), a nod to the Spectral Network (SpecNet), hyperspectral sensing, surface reconstruction, geometric hypersurfaces in gradient-based optimization, and hypernetworks in deep learning. This new breed of hyper-spatial, -temporal, and -spectral surface data are needed to learn new models of radiative transfer, physiology, metabolism, ecology, and ultimately, evolution, making traditional forest inventories look like something from the Stone Age. Machine learning enables learning models from data, with or without prior process knowledge, while edge inference (i.e., real-time prediction for embedded systems) can be used to filter and compress data streams to within communications and disk storage limits, as is often done for drones.
Credit: Adam Erickson
What motivated you to pursue a career in terrestrial biospherics?
Like many kids, I held a deep curiosity for the natural world, which for me were the dark, towering, mist-filled evergreen forests of the Olympic Peninsula. Of course, I wanted to be a paleontologist, an interest that took a technological turn after reading Jurassic Park and subsequently every other Crichton book. I was fortunate to have teachers that furthered this interest at a young age, one for computer science and physics, and another for biology who let me stay after school to dissect specimens. It wasn’t long before I found my calling in research during my undergraduate thesis. While my undergraduate research focused on transportation and energy policy, my (voluntary) master’s thesis focused on watershed management policy. I made this change in research direction after volunteering in ecosystem restoration, where I learned of the fundamental challenge to sustainability posed by land-use and its relation to transportation and energy policy.
Inspired by the work of C. S. Holling at University of British Columbia (UBC) on resilience theory and adaptive management, my thesis suggested methods for building resilient institutions for managing social-ecological systems, which has since grown into a popular concept. Upon completing my thesis in 2011, I sought more rigorous methods for measuring the outcomes of natural resource management policies. This search led me to a remote sensing laboratory for my doctoral dissertation research, in which I demonstrated the first hybrid machine-learning land model. I developed this new class of model in 2015 to simulate the combined effects of changing climate and fire conditions on understory solar radiation given interacting competition, disturbance, dispersal, and regeneration processes. Machine learning allowed me to embed a physical model to accurately approximate a new process not represented by the models. What began as a simple Gedankenexperiment (thought experiment) turned out to be transformative, enabling a wide range of new simulation modeling capabilities, now known as ‘scientific machine learning’. My thirst for knowledge was furthered by the thrill of invention.
On evenings and weekends, I built drones for research and helped to get both the UBC Unmanned Aircraft System (UAS) team and Wingcopter (a drone startup based in Germany) off the ground. I also took a deep interest in computing, artificial intelligence, and genomics, which are related in fascinating ways, and proposed novel deep learning and 4-D spectroscopy research for both terrestrial and human spaceflight domains, the latter intended for NASA Veggie. I sought to extend these concepts to the global scale through my initial postdoctoral research at Max Planck Institute for Biogeochemistry. There, I gave the first presentation on deep learning for Earth system science, specified and tested their first CPU-GPU compute node designed for deep learning and other accelerated workloads, and conducted novel research applying machine learning for radiative transfer model emulation, biomass mapping, and inference of environmental contributions to remote sensing observations based on feature importance.
Subsequently, at Washington State University (WSU), I was the first to suggest hybrid deep learning land models and the blending of individual-based, landscape, and terrestrial biosphere models into new global models, each concept an extension of my PhD research. I also suggested a novel concept to the DARPA Advanced Plant Technologies (APT) program uniquely bridging genetic engineering, terrestrial biosphere modeling, and radiative transfer modeling, treating observation synthesis from land model outputs and subsequent inversions as an end-to-end machine-learning inference problem. The approach highlights an important way forward for the assimilation of data into models through a learning paradigm, using simulators for inductive bias to generalize from ground to spaceborne observations and from past to future. A passion for technology has helped immensely in my scientific pursuits.
Credit: UBC UAS team
Credit: UBC UAS team
What are your future research interests and goals?
My desire is to continue to push the state-of-the-art in the field by improving the representation of land-surface processes in Earth system models and their inference from remote sensing records. While general ecosystem models are improving the representation of tropism and metabolism (i.e., food webs), much remains to be done to improve the geometric realism of the land surface and related terrestrial ecosystem processes. These processes include radiative transfer, evapotranspiration, competition, disturbance, dispersal, and regeneration, the latter underlying the forest response to climate change and thus the global carbon balance.
Large discrepancies exist in the representation of life and its generative process, evolution, without which adaptive biospheric feedbacks, such as the well-known Gaia hypothesis, cannot be represented. Existing models lack not only taxonomic detail (i.e., there are no species, only plant functional types) but also human activity as an emergent property of an evolving biosphere, one that is actively reshaping atmospheric chemistry not unlike oxygen-producing cyanobacteria some 3 Gya. The importance of representing human activity for processes related to wildfire, wildlife, and land-cover change cannot be overstated. This past year (2020-2021), we’ve also witnessed the importance of representing disease ecology, while several other processes remain underserved. These include processes related to mass extinction events that have radically transformed Earth.
Improvements to current models will be critical for engineering a sustainable biosphere against the tide of mass extinctions. While artificial intelligence and genetic engineering provide interesting tools for addressing these challenges, they, too, are a natural part of the Earth system not currently represented in models. Advances in these areas will be critical as we look to apply knowledge of the Earth system to other worlds, whether for terraformation or the search for signs of life and intelligence beyond our solar system. Closing these representation and knowledge gaps will be key in realizing the three-part goal of my research. While the former gap is an engineering problem, the latter is scientific, requiring a blend of approaches and thus collaboration between scientists and engineers. NASA provides unparalleled resources for this type of problem and will thus continue to drive innovation in the Earth and space sciences. For me, doing research at NASA is the realization of a dream.
Credit: Adam Erickson
What research accomplishment are you most proud of?
I am most proud of helping to drive the popularization of deep learning and related hybrid machine-learning land models in the Earth sciences. While deep learning is a form of machine learning in artificial intelligence (AI) based on brain-inspired models of computation, hybrid machine-learning land models blend logical, statistical, and mechanistic (physical or other process) components within a single simulation model. One can think of hybrid models as a data assimilation system embedded within a simulation model, although the learning and inference components can also be accessible through interfaces to external programs. While pure machine learning models are pattern-based and data-driven, hybrid machine-learning models further embed process knowledge or simulators to improve inductive bias and thus generalization, known as ‘forecast skill’ in numerical weather prediction.
Artificial neural networks (ANNs) are often used for their universal function approximation capabilities, allowing inference of a range of non-linear dynamics. Some ANNs are also Turing complete, meaning that they can theoretically represent any program that a computer can. Deep learning literally extends shallow ANNs while enabling unsupervised representation or ‘feature’ learning, obviating the need for 'hand-crafted' or engineered features. Of course, a lot of ingenuity was needed to make this possible, particularly regarding the vanishing/exploding gradient problem and exponential improvements to hardware efficiency in terms of operations per second per watt. Machine and deep learning are particularly useful in a hybrid approach for representing and/or inferring poorly understood processes lacking sufficient theory or for accelerating expensive process models with minimal loss of fidelity.
It is the learning of models from data, process model emulation, and embedding of machine learning within programs (i.e., differentiable programming of which probabilistic programming is a special case) that set hybrid machine-learning models apart from traditional data assimilation. In fact, the entirety of data assimilation can be represented by a subset of Bayesian recurrent neural networks with fixed, rather than learned, priors and update functions. Despite the promise of hybrid modeling, there is a need for greater standardization to ensure that the various Earth system component models can interoperate efficiently as they grow in complexity. This implies the need for new artificial intelligence model intercomparison projects, or AI-MIPs, within the World Climate Research Programme (WCRP) Coupled Model Intercomparison Project (CMIP). This may look more like a data science challenge than a typical model intercomparison project, serving to further democratize climate modeling.
When I began research into deep learning and 4-D hyperspectral vegetation monitoring and modeling (i.e., vegetation dynamics in 3-D space over time) with two computer scientists at Stanford in 2014, there was little awareness of the deep learning breakthrough outside of their field. As with drones at the time, deep learning wouldn’t hit the mainstream in science or society for another year. It was considered obscure and a bit science fiction, not unlike CRISPR and synthetic biology. That anyone would take such interest in this or my work on hybrid machine-learning land models was surprising to me. Being a young scientist, I vastly underestimated the value of my ideas. This taught me to believe in myself and the value of seeking out translational innovations occurring in other fields, particularly computer science and systems biology. Receiving kind words of encouragement from genomics pioneers George Church and Steven Strauss also helped, as many at the time viewed any work mentioning genetic engineering with utter disdain.
I am also proud of my related work on Turing biocircuits – a conceptual approach for biosphere optimization (i.e., engineered sustainability as in goal two) based on biological computation, or the view that living organisms perform physical computation. This work established a host of new research directions in terrestrial biogeochemistry. Importantly, it involved novel proposals for deep learning, symbolic regression (i.e., ‘reverse engineering’ equations from observations), co-processor acceleration, genetic engineering, biological computation, and the combination of information, network, pattern formation, and evolutionary theory (i.e., so-called ‘organizing principles’) in terrestrial biosphere models. While this work was primarily a theoretical contribution, published online in 2015, it is today finding practical application in precision agriculture and autonomous plant growth chambers. It also greatly predates recent work on learning process models from data, general models of vegetation dynamics, and deep learning for Earth system science.
Finally, I am proud of our European Geosciences Union (EGU) 2021 Symposium on advancing terrestrial biosphere models that I led, as well as my presentation of deep learning-based synthetic spectranomics (i.e., inferring hyperspectral and structural information from multi-spectral imagery) at the American Geophysical Union (AGU) Fall Meeting 2020. During my AGU presentation, I also proposed physics-informed deep learning for radiative transfer modeling, deep learning program synthesis, and privacy-preserving networks for training models on distributed protected datasets, the latter to address a common challenge in global mapping. Ensuring that training data are not subsequently leaked by model inversion is a related challenge, for which differential privacy is suggested to safeguard against adversarial attacks. Issues of data and thus model bias have also yet to be addressed.
What science question intrigues you the most?
What is life on other worlds? Must life be limited to a basis in carbon and water per the thermodynamic context of the Earth system? Or can it self-organize from other chemical constituents under different thermodynamic conditions? Intuitively, this seems likely given a wide range of potential elemental and energetic combinations and thus a large phase space of chemical reactions, combined with the indiscriminate ‘tinkering’ nature of evolution. Yet, intuition can only take us so far in science; rigor must close the loop. As a corollary, what can life be? While synthetic biology and simulation modeling allow us to test related hypotheses here on Earth, the view of biological computation may provide new insight into the thermodynamic properties of ecosystems given its intersection with information theory. This may help us to understand life from a systematic view beyond the entropy reductionist views of Schrödinger and Lovelock, individual- and biosphere-based, respectively.
While Earth system models provide an ideal testbed for exploratory work on biological computation related to information theory (i.e., the Kolmogorov or algorithmic complexity of ecosystems and the biosphere compared to other component systems), much remains to be done. A natural extension of this question is the nature of intelligence and its signature within ecosystems across evolutionary timescales. Of course, a far bigger question is why life, intelligence, and the system of evolution that generates both exist at all. Other big questions are (1) why the language of mathematics is universal in describing physical systems, (2) where physical laws may fail, and (3) the true nature of the wave equation, as I tend to support neither the randomness nor the multiverse hypothesis. While the multiverse is not falsifiable, nothing in nature is random, although it is a useful mathematical abstraction.
What advice would you give your younger self?
I have always loved math and I received good marks in it as a kid, but I would tell myself to delve deeper into the subject sooner in life. Every scientific problem can be reduced to a mathematical one given its unreasonable effectiveness in the natural sciences. Then again, deep learning systems based only loosely on theory are working wonders in symbolic mathematics, so maybe that too will be automated. But somehow, aesthetics matter in science, perhaps as a byproduct of our evolved perception of symmetry. The beauty and efficacy of mathematics are as undeniable as that of the emergence of life and evolution from simple physical interactions, each indicative of a broader system beyond our realm of knowledge.
I would also tell my younger self to write some Python scripts (Julia did not yet exist) that invent these things called search engines and social media platforms and maybe an online bookstore named after a tropical forest. I guess then I would never have to worry about research funding. Otherwise, I wouldn’t change a thing, as it was my path that brought me here. I am fond of the saying that one gets luckier the harder that one works.
Credit: Adam Erickson
What do you like to do in your free time?
As time is a precious resource, given our ephemerality and its unidirectional arrow, I prefer to spend time with my family when I am not busy doing research or ‘drinking from the arXiv firehose’ to stay up on the latest in deep learning. Otherwise, I enjoy traveling, the outdoors, and building things in the spirit of Claude Shannon, whether software, computer hardware, unmanned systems, or wetware in the culinary form of Swedish pancake batter. Perhaps unsurprisingly, my best ideas tend to arrive when I am away from the computer and being moderately physically active, as when walking or running. Taking a moment away from the screen is key in solving difficult conceptual problems by seeing the problem with the mind’s eye. Intuition is a powerful tool not only in math but in science, guiding practical and theoretical advances based on a growing foundation of knowledge.
Credit: Adam Erickson
Biography
Home Town:
Aberdeen, Washington, USA
Undergraduate Degree:
International Political Economy, University of Puget Sound, Tacoma, Washington, USA
Post-graduate Degrees:
Master of Community and Regional Planning, University of Oregon, Eugene, Oregon, USA
Doctor of Philosophy in Forestry, University of British Columbia, Vancouver, British Columbia, Canada
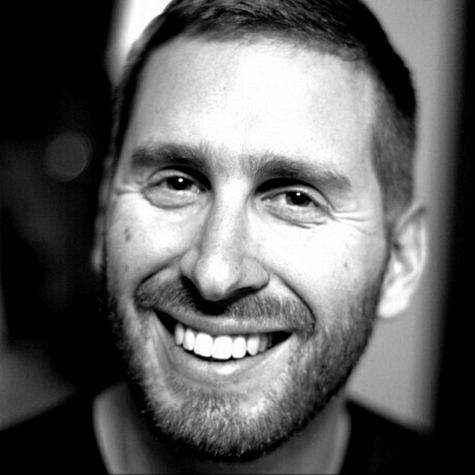
Link to Dr. Erickson's GSFC Bio